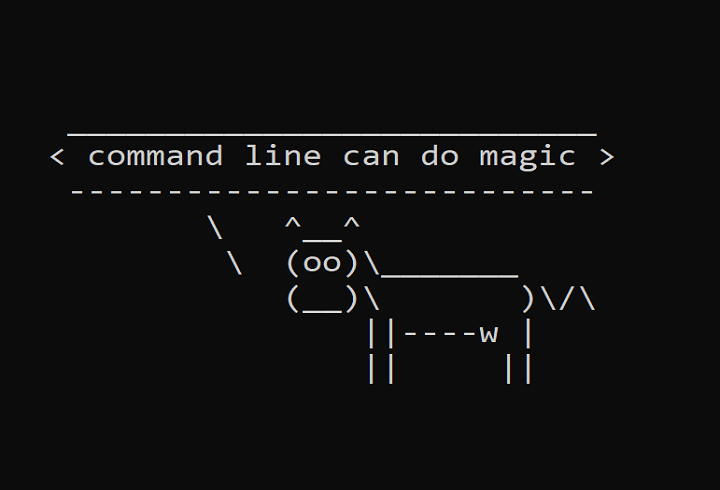
Processing Large Data using compression, data.table and the command line
here we are going to see different ways for processing big data using R and command line
Github Link: https://github.com/MNoorFawi/large-data-processing-with-command-line
first we will generate the data and writing it to disk
set.seed(13) x <- matrix(rnorm(1e7), ncol = 10) write.table(x, file = 'large_file.txt', sep = ",", row.names = FALSE, col.names = FALSE)
our first way to read such large data is to read a compressed version of it
system("cp large_file.txt large-compressed.txt") system('gzip large-compressed.txt') system.time( uncomp <- read.table('large_file.txt', sep = ',') ) ## user system elapsed ## 17.23 0.30 17.64 system.time( comp <- read.table('large-compressed.txt.gz', sep = ',') ) ## user system elapsed ## 9.34 0.03 9.44
a big difference in time need to execute both reading commands
and yet there’s another great tool to read such large files DATA.TABLE
library(data.table) system.time( funcomp <- fread('large_file.txt', sep = ',') ) ## user system elapsed ## 0.50 0.07 3.47 system.time( fcomp <- fread('< large-compressed.txt.gz gzip -d', sep = ',') # gzip -d is a command line tool to unzip gzip files ) ## user system elapsed ## 0.41 0.05 0.12
there’s almost no difference between freading compressed or uncompressed data. Both ways are better than regular read.table even with compressed data.
data.table::fread also supports some command line functionalities which we’re going to explore later on.
for example
# we can exclude any rows with a negative value positive_only <- fread( "< large-compressed.txt.gz cat | gzip -d | grep -v '-'", sep = ',') # get the number of rows, which will be a data.table object fread('< large-compressed.txt.gz cat | gzip -d | wc -l') fread('< large_file.txt wc -l') ## V1 ## 1: 1000000
Data Processing with Command Line Tools
the command line has very useful tools that are great in dealing with large and even very big data and parallelism as well.
to get the full benefits from the command line we need to have some tools installed first:
- if we’re using Windows we need to setup cygwin tools, gnuwin/awk, etc..
- other tools like “csvkit” which has great functionalities to deal with data we can install it using pip install csvkit
- there’s also a great toolbox for doing data science with the command line which is DataScienceToolbox developed by Jeroen Janssens author of the great book “Data Science at the Command Line” we can either install his magnificent virtual environment or download the tools git clone https://github.com/jeroenjanssens/data-science-at-the-command-line.git and put their folder in the path #### then we can use these tools in Windows using cygwin terminal or Git Bash.
let’s get down to business first, I want to compare between the time need to read and extract only the rows with all positive values using Command Line and R
# we have "comp" variable which took almost 25 minuts to get read system.time( comp[-which(comp < 0, arr.ind=TRUE)[,1],] ) ## user system elapsed ## 0.57 0.03 0.60 # add this time to the time needed to read it
using the command line
time < large-compressed.txt.gz cat | gzip -d | grep -v '-' > output.txt #$ real 0m2.124s
such a huge difference.
now it’s time to explore more tools and to see what else we can do with the command line
# here we read the zipped file then we exclude any negative value then we choose only the rows where columns 7 is greater than or equal to 2 and column 5 is greater than 1 then we get the number of rows < large-compressed.txt.gz cat | gzip -d | grep -v '-' | awk -F, '($7 >= 2) && ($5 > 1)' | wc -l #$ 21 # here we read the data and get the rows that have any negative value # then we select rows where column 1 >= 2 or column 2 < 0 # and return columns 3, 4, 5+6, 7*8 and naming the columns a, b, c, d # and showing the first 10 rows including the header with a nice look < large-compressed.txt.gz cat | gzip -d | grep '-' | awk -F, '($1 >= 2) || ($2 < 0) {print $3","$4","$5+$6","$7*$8}' | header -a a,b,c,d | head | csvlook
a | b | c | d |
---|---|---|---|
0.263 | 0.248 | -0.079 | 0.280 |
0.605 | 0.902 | 0.549 | 0.207 |
-1.124 | 0.853 | 1.471 | -0.183 |
-0.578 | -0.462 | 0.760 | 0.706 |
-1.108 | -0.396 | 1.091 | 0.115 |
0.939 | -0.342 | -2.619 | 0.042 |
-2.101 | 2.659 | -4.484 | -0.141 |
0.922 | -0.570 | -1.287 | -1.327 |
1.069 | -1.227 | 1.076 | -0.296 |
# we can also write the output to a file then read it with R and do our analysis on it being much smaller < large-compressed.txt.gz cat | gzip -d | grep -v '-' | awk -F, '($7 >= 2) && ($5 > 1)' > smaller_file.csv #fread('smaller_file.csv', sep = ',') # we can also use the functionality of SQL within command line < large-compressed.txt.gz cat | gzip -d | csvcut -c 7,8,9,10 | header -a g,h,i,j | csvsql --query 'SELECT SUM(g) AS sum_a, AVG(h) AS mean_h, MIN(i) AS min_i, MAX(j) AS max_j FROM stdin' #$ sum_a,mean_h,min_i,max_j #$ 604.6188991213197,-0.0003848574020195104,-4.98809626002303,4.68823847832136
as we saw, Command Line can be of very great use when it comes to processing large files. and we can combine it with the analytical power of R to gain better performance. for example we can preprocess data in command line then reading the processed data in R and do our analysis. finally I want to recommend reading https://www.datascienceatthecommandline.com/ book …
Leave a Reply